
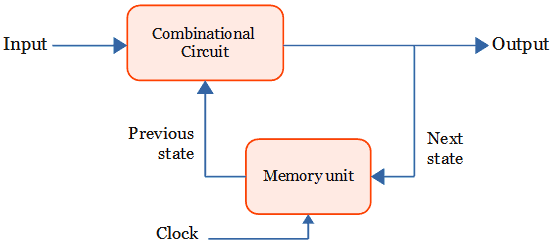
It accepts the configuration of the model. It further helps you to create a new model. It accepts the representation of the model in the form of a JSON string. This representation is in the form of a JSON string. It returns the representation of the model. It provides the configuration of the model in the form of the dictionary. It provides you a list of all the weights tensor as a NumPy array.
SEQUENTIAL MODEL FULL
It provides you a summary of full information about the model and the layer. It helps you to create a summary of a model. This method is useful in returning the list of all the output tensors of a model.

It provides you the list of all the input tensors in a model. It is the list of the input tensors of the model. It informs you about the layer that comprises a model. This method returns all the layers of the model as a list. These methods help you to get the model information. To access the model, Keras provides a ton of methods. Accessing and Serializing the Keras Model 3D layers have input_dim and input_lenght to specify the input shape of the model. For 2D layers like Dense, there is input_dim argument. You can pass this argument to the very first layer.Ģ. To specify the input shape to the layer, there is a number of methods available. Because the output of the first layer becomes the input of the sun-sequent layers automatically.ġ. You need to specify the shape of the first layer only. It is necessary to specify the shape of the input to the layer. To use add() method, you initially need to create a layer using API then pass the layer through the add() method. You can add any layer easily by the add() method. from keras.models import SequentialĪdding a layer in the Sequential Model is very easy. You have to pass a list of instances to the constructor. You can create a Sequential model by calling the Sequential() method. Most of the ANN’s use the Sequential API Model. The flow of data is continued until the data reaches the final layer. In this model, the data flow from one layer to another layer. According to its name, its main task is to arrange the layers of the Keras in sequential order. It is a linear stack of methods that groups a linear stack of layers into a tf.keras.Model. There are two types of Models available in Keras: The Sequential model and the Functional model. These represent the actual neural network model. Confusion matrix is drawn to show the correctly classified and misclassified samples.Keeping you updated with latest technology trends, Join TechVidvan on Telegram Keras Models The accuracy of the training set is 100 percent and the testing set is 96.7percent. The extracted features are fed into the sequential keras model which recognizes the plant species. One hot encoding is also applied onto the target values to optimize the results of recognition. From these samples the features like shape, color, texture, corners are extracted. Five different plant species are chosen as samples among the Indian species, namely OcimumTenuiflorum, Sansevieriatrifasciata, Chlorophytumcomosum, Azadirachtaindica, Aloe Vera. Keras is a widely used deep learning framework which is employed in this work. This is one of the accepted technologies that automatically extracts features, processes them and yields the best results. Deep learning is a subset of machine learning.

Various researchers have applied different approaches to recognize the plant family. Plant recognition is a very important task to segregate the huge amount of floras which belong to various categories. Lots of varieties of species are beneficial to the human's life.
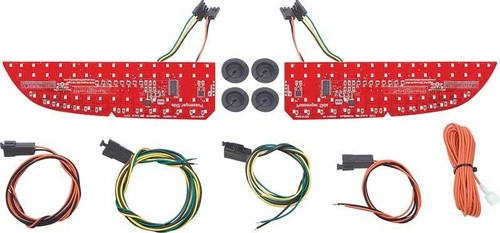
There are huge varieties of floras in the world.
